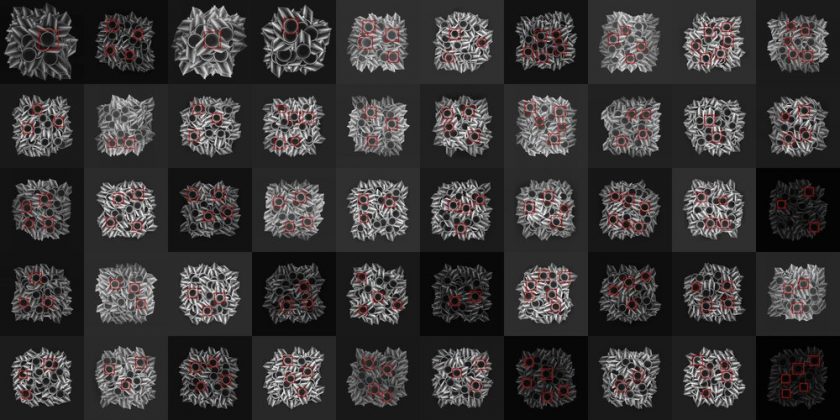
EDI develops deep learning-based (CNN, RNN, GAN) solutions for various applications. We are most experienced in working with computer vision tasks – using camera data to detect vehicles and their parameters, recognize number plates, identify people, distinguish among crops and weeds, detect which objects in a pile can be picked by an industrial robot, classify objects on a production line and more. However, the machine learning approach can be similarly used for processing of data from other kinds of sensors.
In video and image processing, we are offering to take on image classification and segmentation, object localization, detection and recognition tasks. To successfully apply the deep learning methods, one needs appropriate annotated data. The acquisition of such data is often long and labor-intensive. Therefore EDI is developing technologies to deal with the data problem. Depending on the task, EDI proposes several approaches:
* Semi-automatic labeling, in which our software eases and speeds up the labeling of the data;
* Generation of synthetic data using 3D modeling;
* Generation of synthetic data from several 2D images;
* Generation of realistic data using image translation (CycleGAN).
Training of deep models and generation of data is accomplished using EDI HPC server which has 12 NVIDIA Tesla k40 accelerators.
Publications:
Roberts Kadiķis